More Information
Here, you’ll find more information about the paper, models, and equipment. Detailed information about the code and how to use it is in the repository.
Paper’s Results
Meta-analysis comparison of PSG- and CSG-based sleep staging
The main takeaway from the paper is that our model performs equivalently to human-scored PSG. To make this comparison, we performed a meta-analysis using the data from 11 human-to-human studies to get the overall and stage-specific kappas that can be expected for humans. Then, we compared our results against the human performance.
Forest plots for overall and stage-specific kappas and our model’s performance. At the top are the forest plots for the random-effects estimates for overall and each stage-specific Cohen’s kappa (κ) for human-to-human inter-rater agreement on PSG. We list the source inputs chronologically, with each mean kappa and CI to the right. Per convention, the gray square for each represents their weight. The unfilled black diamonds represent the random-effects estimates (width representing each estimate’s 95% CI), and the whiskers extending from the diamonds represent the 95% PIs. The black vertical dotted lines also indicate the random-effects estimates. At the bottom are the overall and five-stage kappas of our primary model based on single-lead ECG evaluated on our testing set. All non-inferior comparisons using a t-test are significant (i.e., noninferior; *p < 0.025)—except for N3 (not non-inferior).
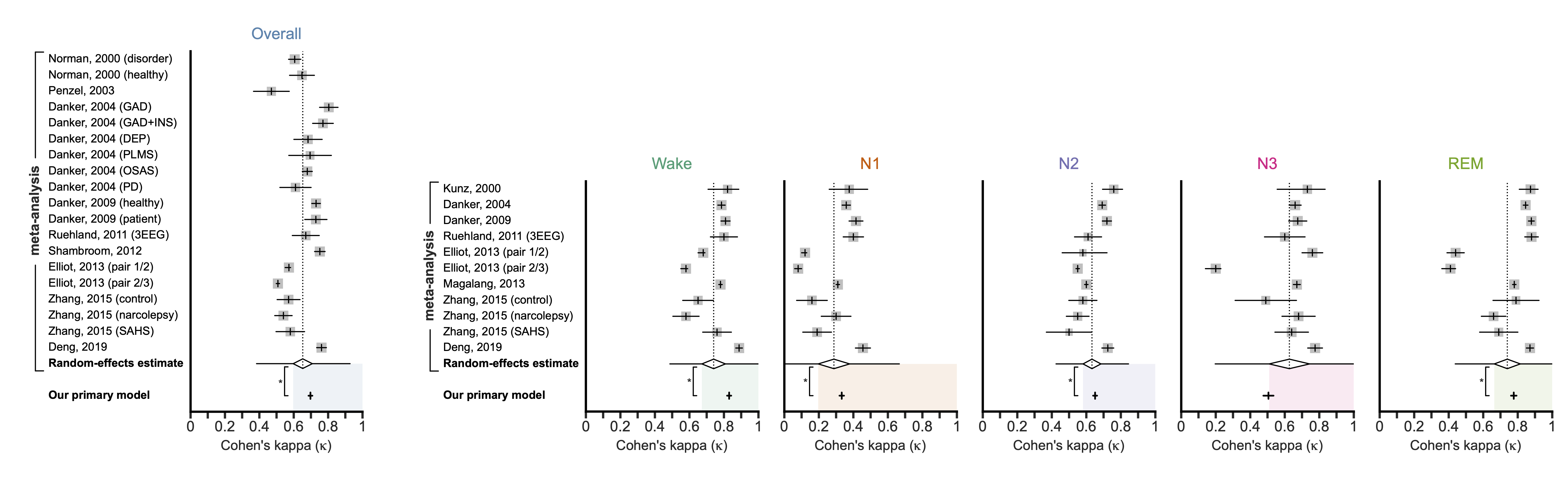
Available Models
There are currently three models in the repository that anyone can use to score their own ECG data:
- Primary model
- This model is designed to take an entire night’s worth of sleep and score it all at once.
- Real-time model
- This model is designed to take just the data that has been recorded up till “now” and score it all at once. As each new 30-second epoch of data is recorded, the model can be run again.
- NEW Primary model without demographics
- This model was not in the paper, having been trained just recently.
- The model works the same way as the primary model, except you do not need to provide the demographics (age and sex) of the subject.
- There is a slight (<1%) performance impact, as measured with Cohen’s kappa, compared to the original primary model.
ECG Equipment
Unfortunately, there are few commercial ECG recording devices on the market right now. I am only aware of two devices that could possibly work and be worn comfortably while asleep. However, I have not personally tried either yet, so I cannot vouch for them. (Blog update: I’ve begun testing them.)
- Movesense
- The sampling rates are: 125, 128, 200, 250, 256, 500, and 512 Hz.
- Polar H10
- The sampling rate is: 130 Hz.
However, if you are using clinical or research equipment to record ECG there are a few important details to keep in mind:
- The recording should be of Lead I (limb lead across the heart). See ECG Lead positioning for the proper locations of the “RA” and “LA” electrodes.
- The sampling rate should be a minimum of 125 Hz. Higher is better, with the “optimal” rate for the network’s input being 256 Hz.
- The sampling resolution should be a minimum of 16 bits. Higher is better.
References
Our paper
If you find this repository helpful, please cite the paper:
- [1] Adam M. Jones, Laurent Itti, Bhavin R. Sheth, “Expert-level sleep staging using an electrocardiography-only feed-forward neural network,” Computers in Biology and Medicine, 2024, doi: 10.1016/j.compbiomed.2024.108545.
- Contact details provided on this website and inside the paper.
- Preprint version of paper @ medRxiv
- There were some large changes between the preprint and the final, published, version (mainly revolving around adding a large meta-analysis). However, the network and testing set results are the same.
Additional works cited
- [2] T. Wang, J. Yang, Y. Song, F. Pang, X. Guo, and Y. Luo, “Interactions of central and autonomic nervous systems in patients with sleep apnea–hypopnea syndrome during sleep,” Sleep Breath, vol. 26, pp. 621–631, 2022, doi: 10.1007/s11325-021-02429-6.
- [3] T. Penzel, J. McNames, P. de Chazal, B. Raymond, A. Murray, and G. Moody, “Systematic comparison of different algorithms for apnoea detection based on electrocardiogram recordings,” Medical and Biological Engineering and Computing, vol. 40, no. 4, pp. 402–407, Jul. 2002, doi: 10.1007/bf02345072.
- [4] H. Hilmisson, N. Lange, and S. P. Duntley, “Sleep apnea detection: accuracy of using automated ECG analysis compared to manually scored polysomnography (apnea hypopnea index),” Sleep Breath, vol. 23, no. 1, pp. 125–133, Mar. 2019, doi: 10.1007/s11325-018-1672-0.
Readers and Mentions
Expert-level sleep staging using an electrocardiography-only feed-forward neural network